For 48-692: Shaping Daylight
By Vishal Vaidhyanathan and Afshan Rehman
By Vishal Vaidhyanathan and Afshan Rehman
This study proposes a simple yet efficient methodology for conducting qualitative studies remotely and on a mass-communication level using web-based Virtual Reality (VR) technology.
The value of daylight influence in various aspects of architectural design and spatial cognition have been studied and analysed. It has been established that daylight drives a powerful combination of qualitative and quantitative effects on a space, and that studies of daylight performance of a space are necessitated to inculcate both. However, for thorough survey-based perceptual evaluations of day-lit spaces – especially VR-based immersive surveys, access to physical survey subjects is required, unlike regular web-based surveys that can be mass-communicated. Through the lens of an executed remote web-based immersive survey platform for perceptual study of a day-lit space, this study aims to demonstrate the potential of this method as an efficient mode of perceptual daylight study without access to survey subjects by analysing daylight quality of the space on two metrics - Light quality and Spatial Cognition.
Key-Innovations:
- This research removes the potential drawbacks of not having remote surveys possible – like inability to conduct such studies during pandemic situations.
- The real-time photorealistic immersive VR environment survey allows the users to abate any kind of cognitive biases that may arise in perceptual surveys.
- A large sample set of subjects can take the immersive survey remotely and their corresponding data can be recorded online in cloud.
- This research also introduces gamification – a survey methodology which makes mundane activities more interesting and ensures psychological neutrality and subject involvement.
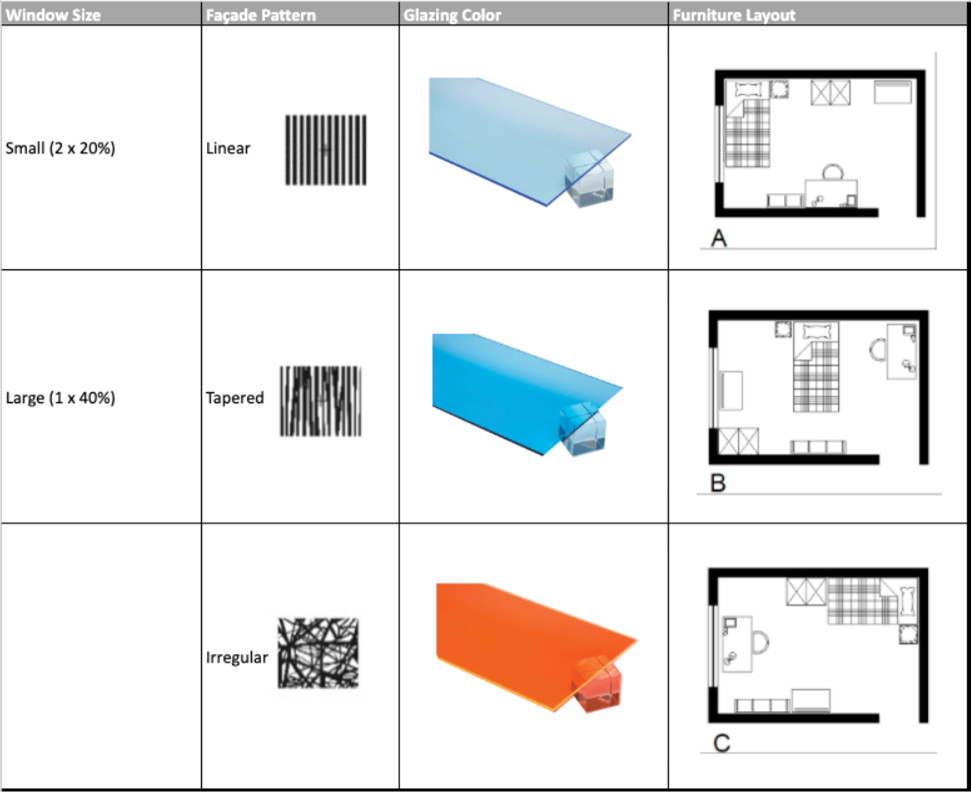
Variables and hierarchical parameters in the web-based interface that are chosen for this study
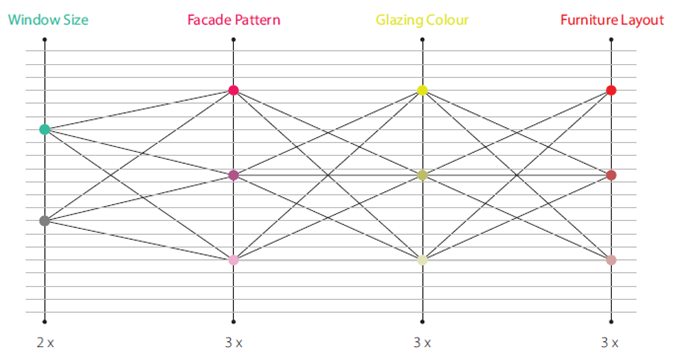
Parallel Plot Chart of 54 variable parameter combinations
For the purpose of this study, a dorm room in Shady-side, Pittsburgh was chosen. An initial 3D model of the room was made in 3DS MAX to understand the dorm spatially. The room is then imported to Rhino3D and a complete daylight simulation is done in ClimateStudio.
Isometric View 3D model of selected space in 3DS Max
Perspective View 3D model of selected space in 3DS Max
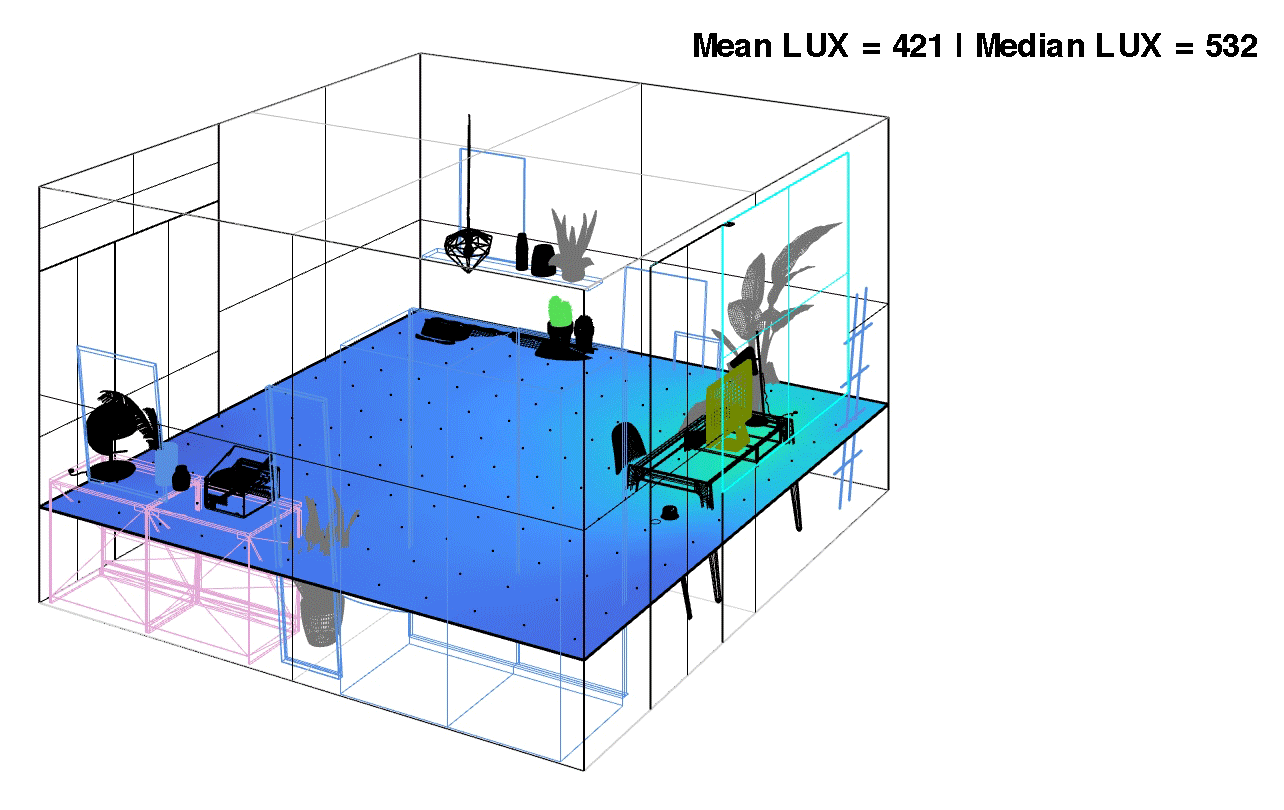
Quantitative Daylight Factors of the space
Weather Data: Pittsburgh.Intl.AP.725200_TMY3
Cardinal Point: Window opening faces east
Room Size: 4.67 m X 4.67 m
Cardinal Point: Window opening faces east
Room Size: 4.67 m X 4.67 m
Materials:
- Floor – Wood Walnut (Rvis – 16.2%)
- Door – Wooden Door (Rvis-5.7%)
- Window – Double IGU Blue Tvis 38%
- Wall – Dupont White 106 (Rvis-85.8%)
- Ceiling – Ceiling LM83 (Rvis-70.0%)
- Floor – Wood Walnut (Rvis – 16.2%)
- Door – Wooden Door (Rvis-5.7%)
- Window – Double IGU Blue Tvis 38%
- Wall – Dupont White 106 (Rvis-85.8%)
- Ceiling – Ceiling LM83 (Rvis-70.0%)
Sensor:
- Sensor spacing – 0.4m
- Sensor offset – 0.7m
- Occupied Hours – 8am to 6pm with 60 minutes DST
- Sensor spacing – 0.4m
- Sensor offset – 0.7m
- Occupied Hours – 8am to 6pm with 60 minutes DST
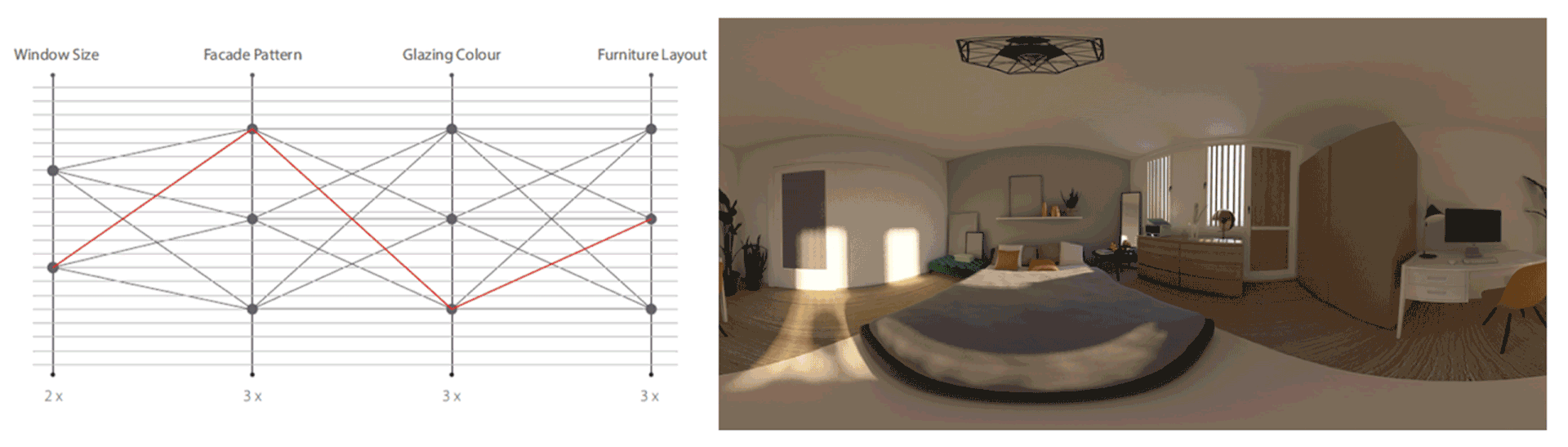
Examples of 3 trigger variables
A complete interactive virtual environment of the spatial context is developed with real time variable control and parametric freedom of choice. The virtually constructed daylit space is programmed to emulate real life sunlight with respect to time. While all the elements that are composed within the virtual immersive space are static, the trigger variables and their expanded iterations/typologies that were identified in the pilot study are made parametric, hence offering a freedom of choice and control to immersed survey subjects. An interactive user interface is designed to allow subjects to identify trigger variables while exploring the virtual space. A slider is provided to those trigger variables for the subjects to change until the space attains perceptual comfort.
Final Interface of the web-based survey
The tool is still under development where the recorded data is dimensionally reduced into palpable formats to be fed into a simple architecture Artificial Neural Network. The ANN identifies – through logistic regression, or classification algorithms like K-means clustering – to approximate the context-based trigger variable parameter function. The ANN aims to identify and learn the trend by which subjects choose a particular trigger variable parameter based on the spatial context.
The ANN will then be trained on the recorded survey data as the training dataset. The model is then tested for training accuracy and will be tuned until an accuracy of at least 90% is achieved. The trained model is then built and can be used for multiple applications of design assistance, by coupling with different technological platforms and concepts like Augmented Reality (AR), Generative Systems, etc.